Quality assurance with ML/AI Artificial Intelligence
We work intensively with machine learning – a sub-area of the field of artificial intelligence.
Coming from the field of classic Image processing, our Machine Learning also revolves around the processing of image data.
We can also realize more complex tasks that require the combination of classic algorithms and machine learning or the combination of different data sources (analysis of several images of an object, integration of additional sensor data or user input).
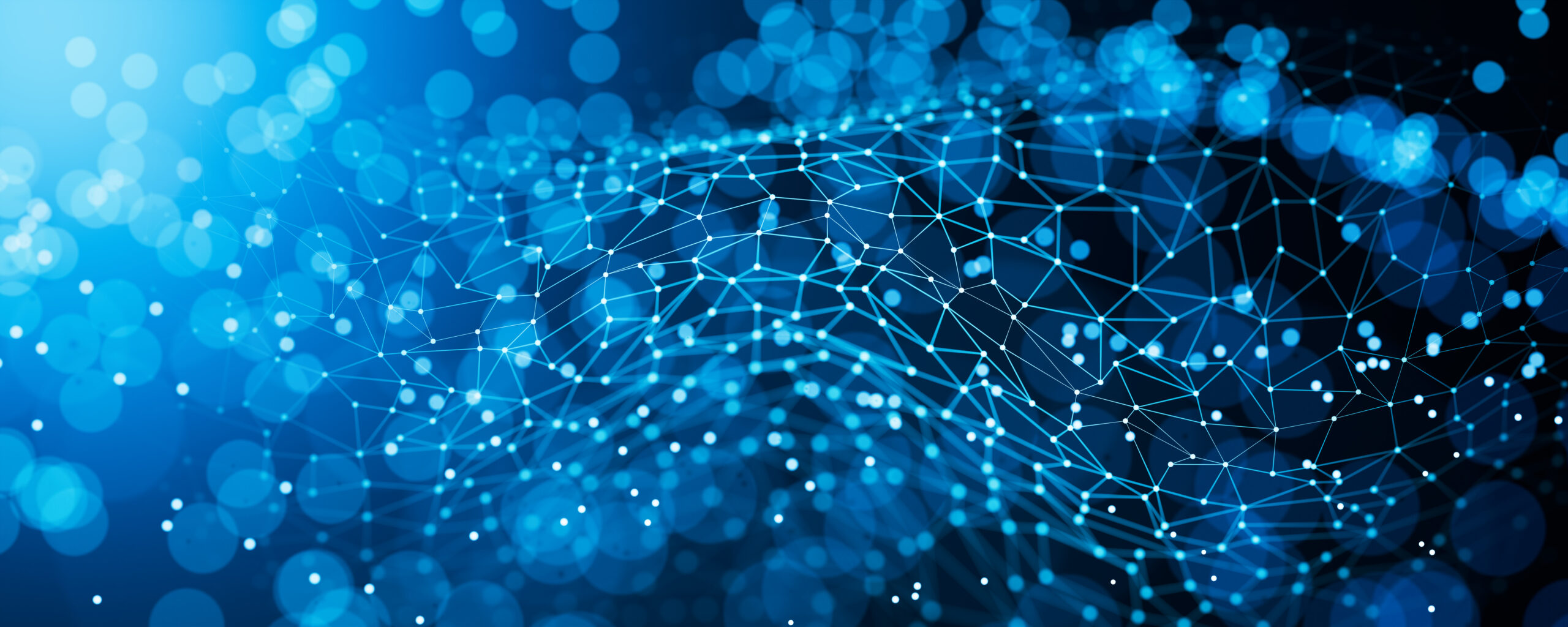
- Classification
- Multimodal data processing
- Object detection & localization
- Anomaly detection
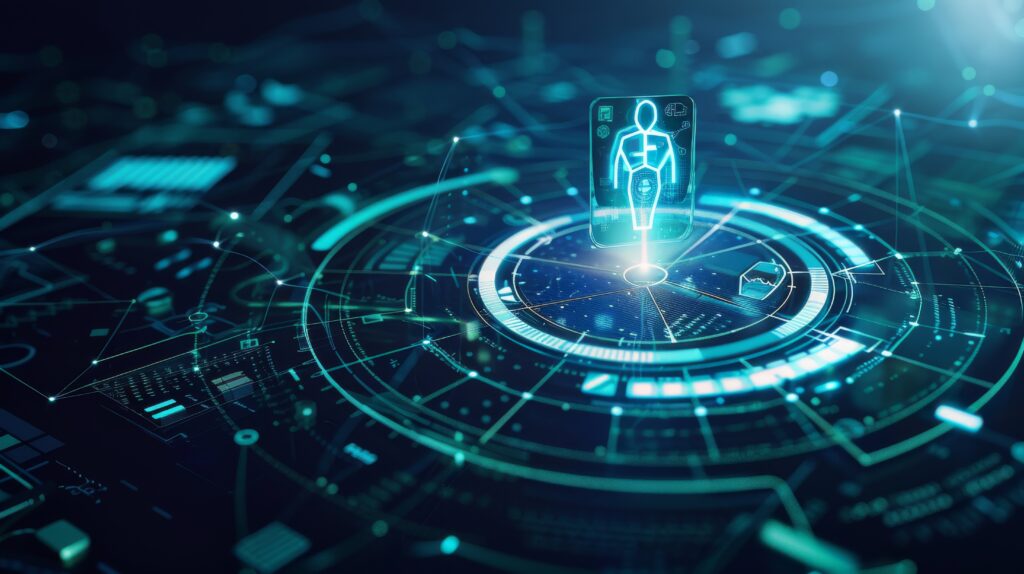
Classification
- Differentiation into any classes (e.g. [good , bad], or [good , error type 1 , error type 2]).
- Training based on a large amount of training data for all classes.
- Detection of very subtle differences possible.
Multimodal data processing (“extended classification”)
- Differentiation into any classes (e.g. [optimum quality , average quality , rejects]).
- Training based on a large amount of training data for all classes.
- Input data from different sources is combined, e.g. several camera images, additional product information such as color, shape or weight or user input.
- Combination of different algorithms possible.
- Recognition of differences, which is only possible from the combination of different data. Inspection of an entire product is possible (e.g. the entire surface through several images which are evaluated together).
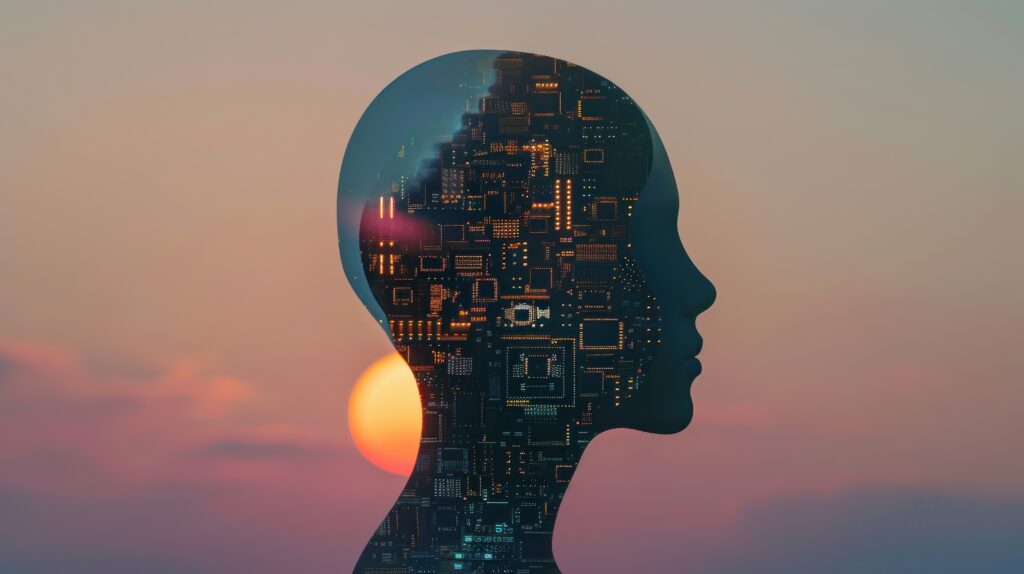
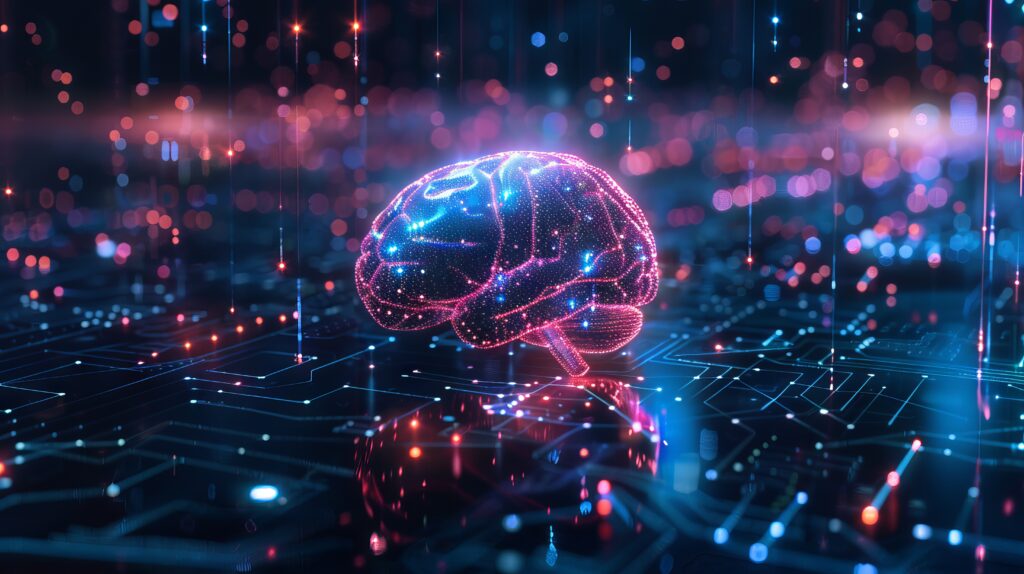
Recognition & detection
Object detection & localization
- Recognizing objects, including determining their position.
- Training based on training data of all objects.
- Among other things, this enables presence checks or the counting of objects.
Anomaly detection
- Differentiation between “normal” and “anomalous” data.
- Training is usually carried out with a small amount of normal and abnormal data. If not otherwise possible, training on the basis of 100% normal examples is possible.
- Depending on the degree of anomalies, medium to good detection accuracy is possible.
Frequently asked questions (FAQs)
Was ist der Unterschied zwischen klassischer Bildverarbeitung und dem Machine Learning Ansatz?
In classic Image processing, humans determine certain evaluation features and program a corresponding rule-based algorithm.
The use of machine learning automates the selection of suitable evaluation characteristics. In other words, algorithms use training data to learn which features are important for an evaluation. This makes it possible to solve complex tasks in which a large number of features have to be combined and taken into account.
Welchen Vorteil hat der Kunde durch Machine Learning?
Machine learning provides new ways of tackling tasks that were considered unsolvable or too cost-intensive a few years ago.
Welche Aufgaben können mittels Machine Learning gelöst werden?
The strengths of machine learning are particularly evident in the following areas:
- Detection of defects (of unknown severity)
- Classification of objects with variable geometry
- Quality assurance – evaluation of products
(similar to the manual process, learning is based on limit samples [Trainingsdaten] )